Big Data and Artificial Intelligence in Credit Risk Management
A while ago, input data were just the companies’ public information (e.g. balance sheets), but thanks to the quick evolution of technologies, the amount of information analyzed increased, and Big Data required to be studied through appropriate methods.
Today's computational resources, which are constantly expanding, allow us to relate the most diverse elements, among which we will link significant industry trends, geographical position, news, social networking behavior, management and members relations, etc.
How do you relate this information? How do you analyze them and which are their relevant connections within the assessment of a probability of default?
Mathematical modeling
At the beginning of Big Data analysis there is a numerical mathematical model, a set of coded rules in a precise procedure, which describes the probability of a phenomenon’s evolution, based on input data and returning output data. The more precise and accurate the answer, the more powerful the computational modern technology (it must process all the data and monitor it constantly).
In a Credit Risk Management service, this mathematical model gives us the opportunity to develop a real-time probability of default: whenever one or more of these input information changes, it is reclassified inside the engine and it engages (and possibly modifies) the probability of default of the given entity.
Let’s now introduce Artificial Intelligence and Machine Learning: in the past anyone who wanted to analyze Big Data had to create a pre-configured model and arranged the parameters with an automated methodology, in order to get the relations, now these models are auto-adapting algorithms.
One of the most used Machine Learning technologies is Neural Network, a mathematical model consisting of artificial "neurons" used to solve problems. Without pre-defined models, the neural network processes the information, adapting itself to rebuild the model standing between input and output.
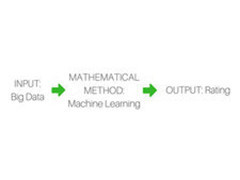
Analytics
However, one of the main factors in Credit Risk Management is to determine the dynamics that caused the rating evaluation and to integrate this information to an industry and geographic level. To do this, the use of Analytics is critical.
To further understand how and why we get the output results, and which the relationships between input and output are, we adopt a range of statistical methodologies’ graphical representation. One of these is the Self Organizing Map, which sorts and groups homogeneous elements within a set of data.
All these methodologies come from statistics: this means that, although highly accurate, they study a massive information general trend, and may lose the singular position. And, because of this, we obtain a proper general evaluation, possibly mistaken on the individual case. The credit rating therefore uses this statistical study, but as we can’t afford to lose the single position, which is the most interesting, we need to have a further step: validating the single position.
To overcome this difficulty, which usually means passing through a human analyst, modefinance has developed the innovative MORE methodology: a mathematical model which adapts the most advanced technologies to a financial analyst normal behavior, able to give a reliable, accurate and certified output.
to learn how to financially
evaluate partners,
competitors and customers